Use AI, Machine Learning to Take Variability Out of Manufacturing
Variability has always created insurmountable challenges for manufacturers. However, in the last decade, impact has been more painful due to a lack of experienced workforce. By leveraging technological advancements, particularly in AI, manufacturers can gain a transformative competitive advantage in both cost reduction and workforce development.
Manufacturing productivity is affected by billions of changing variables every single day. Variability from operators calling in sick, to weather events creating humidity, or from fluctuations in supplier materials. This variability can translate into billions of dollars lost over time.
Fortunately, with technological advancement in the monetization of data, more of these variables are being addressed and manufacturers are seeing gains that were previously out of reach.
How Variables Impact Manufacturers’ Core KPIs
Manufacturers are always aiming to make more with less, while aiming to meet quality specifications. Introducing variability complicates this by making an ideal scenario nearly impossible to achieve and replicate. What’s worse, variability is present and compounds in every stage of the production process, including what product is being made and the operators on shift. This creates a multivariant problem that is difficult to proactively identify, analyze and correct, particularly before it costs the manufacturer time, material or employee resources.
Because of this, variability in the production process affects core key performance indicators (KPIs) in the following ways:
- Availability: Variability in processes, materials or operators can cause unplanned downtime and maintenance issues. What’s worse, because of the amount of sources, the causes and reasons for unplanned downtime are hidden and not categorized. This makes it impossible to correct and prevent downtime for the future. According to GE, oil and gas manufacturers who take this reactive approach to variability in downtime see an average of 8.43% unplanned downtime, while those who invest in predictive see closer to 5.42%. This difference could mean millions in productivity loss.
- Performance: Line speed is often set by machine specification, financial models or even hunches. This creates a gap between what is repeatedly achievable without risking quality and what the average line speed is. It’s in the company's best interest to understand what is achievable line speed at a product/line combination level, and incentivize operators to run lines as close to that target as possible (usually the 75th percentile).
- Quality: Quality specification must be over indexed to ensure quality doesn’t fall under minimum requirements if any part of the production process is affected by variability. An example of this is giving away 5% of material in wall thickness with product.
Of course, this does not address all sources of performance loss due to variability, but these are high impact sources. Addressing variability in each of these areas will result in improvements in the company’s bottom line.
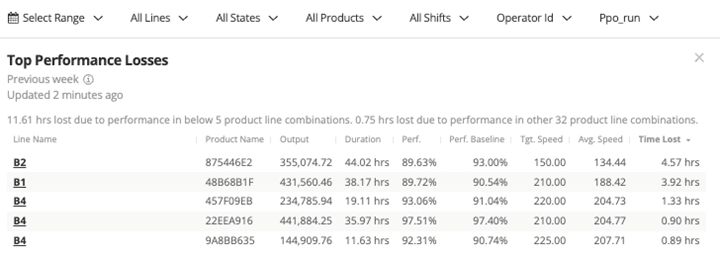
Variance in line speed against achievable performance can cause hours of lost productivity time. Photo Credit: Oden Technologies
Variability Costs Have a Direct Correlation With Workforce
Historically, manufacturers have depended on experienced operators and other frontline employees to reduce the negative impact of variability. These experienced staff members know how to react and compensate to reach high productivity. We commonly see that the top 10% of operators run their lines 22% faster than average, with the bottom operators running 10% slower.
With the large majority of manufacturers facing workforce challenges, depending on tenured expertise is no longer possible.
Fortunately, artificial intelligence (AI) and machine learning have made massive strides in the last few years to enable the front line to make informed and gainful decisions, regardless of tenure.
What AI Is Good At
AI doesn’t do everything, and manufacturers shouldn’t expect it to come in and solve all of their problems. AI is good at:
- Quickly cleansing large data sets
- Analyzing patterns in large datasets
- Quickly optimizing for quantifiable goals
AI can, in real-time, analyze the billions of intersecting data points created by the manufacturing process and provide the most important information and patterns to frontline teams. This can greatly decrease, or even eliminate, the need to rely on hunches and experience to make effective decisions.
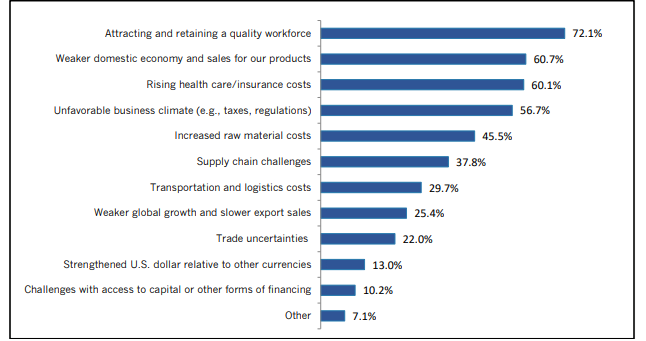
Primary business challenges manufacturers faced in Q3 of 2023, based on research conducted by the National Association of Manufacturers. Workforce issues were tops on the list. Note: Responses exceed 100% because respondents were permitted to check multiple responses. Photo Credit: NAM 2023 Third Quarter Manufacturers’ Outlook Survey
AI Use in the Real-World
A real-world example currently being used by several manufacturers is the use of AI-generated, prescriptive process setting recommendations that most align with organizational goals. To an operator, they see what process settings they need to implement during a run, what the predicted quality would be from those process settings, and what the expected material or cost savings will be. These process setting recommendations are automatically adjusted given current constraints, so they greatly differ from a traditional recipe guide.
How it works:
- Production and process data is cleansed, joined, contextualized and enriched in real-time through a scaled data architecture and models
- Predictive quality models match quality outcomes with current variables, and identify stable periods from which to source process settings
- Stable periods are matched against quality targets and configured constraints, such as line speed limits.
- The most cost- or output-effective grouping of process settings are identified and surfaced to operators.
All of this happens in real-time, so operators aren’t waiting on analysts or engineers to adjust based on changing situations or new information. This use of AI has enabled new operators to execute like they are long-tenured staff. Using this system, a large manufacturer saw a 5% reduction in related material and energy costs while increasing line speed by 3%.
“The Process AI operator interface has allowed the operator to see in real-time how they are adding value when they are making efforts in reducing cost while giving them comfort that they are not going to be off quality (by) utilizing the predictive quality models.” - Senior Process Engineer
Ultimately, this is reducing variability in the process by bringing operator-dependent processes up to a higher standard, creating real-time adjustments for unforeseen events or changing variables, and filtering out process settings that won’t proactively meet quality standards.
Prescriptive process setting recommendations based on real-time conditions and predicted quality can increase every operator’s capability. Photo Credit: Oden Technologies
Preventing Garbage In, Garbage Out
AI greatly depends on clean and relevant data from which to source. To gather this data, many manufacturers are investing in a unified namespace, data warehouse or data lake. However, many companies are underestimating, or overlooking, the unique challenges around preparing manufacturing data to be useful.
In fact, according to LNS, data quality issues are the number one challenge of analytics initiatives in manufacturing. Data analysts, engineers and scientists spend as much as 70% of their time and effort on data cleaning and preparation.
In plastics, it’s typical to need to process over 5 million data points per line every day to get the information needed for AI. These data points need to be aggregated, windowed, custom calculated, enriched and any outliers specially handled. In addition, stable periods need to be identified so that productivity gains are optimized for portions of a run, not just the run as a whole.
Given that AI needs real-time data to be able to create prescriptive recommendations for the front line based on current conditions, building out the data warehouses or data lake structures needed for AI to be productive is a large project on its own. This is why it’s important to work with a partner, or make a large team investment, that understands the entire process, from data consolidation to prescriptive instructions.
About the Author: Willem Sundblad is the co-founder and CEO of Oden Technologies, a company empowering manufacturers to make more, waste less and innovate faster through manufacturing analytics and AI. In his spare time, he is a contributing writer to Forbes.com and takes every chance he gets to enjoy the Green Mountains of Vermont.
Related Content
New Technology Enables ‘Smart Drying’ Based on Resin Moisture
The ‘DryerGenie’ marries drying technology and input moisture measurement with a goal to putting an end to drying based on time.
Read MoreProcessing Megatrends Drive New Product Developments at NPE2024
It’s all about sustainability and the circular economy, and it will be on display in Orlando across all the major processes. But there will be plenty to see in automation, AI and machine learning as well.
Read MoreReal-Time Production Monitoring as Automation
As an injection molder, Windmill Plastics sought an economical production monitoring system that could help it keep tabs on its shop floor. It’s now selling the “very focused” digital supervisor it created, automating many formerly manual tasks.
Read MoreSystem Offers 'Lights Out' Mold-Channel Cleaning & Diagnostics
New system automatically cleans mold-cooling lines—including conformal channels—removing rust and calcium, among other deposits, while simultaneously testing for leaks, measuring flow rate and applying rust inhibitor.
Read MoreRead Next
For PLASTICS' CEO Seaholm, NPE to Shine Light on Sustainability Successes
With advocacy, communication and sustainability as three main pillars, Seaholm leads a trade association to NPE that ‘is more active today than we have ever been.’
Read MoreLead the Conversation, Change the Conversation
Coverage of single-use plastics can be both misleading and demoralizing. Here are 10 tips for changing the perception of the plastics industry at your company and in your community.
Read More