The Fundamentals of Artificial Intelligence and Machine Learning and Their Application to Injection Molding
As AI and ML continue to develop, they can eventually play a role in helping molders predict more accurately and, perhaps someday, model very close to the actual process results.
There is a lot of buzz about Artificial Intelligence (AI) and Machine Learning (ML) these days, as seemingly everyone is talking about and trying to use them in some form to make the systems better. Despite the current buzz, these techniques have been around for a very long time in some form or another.
Back in the early 1990s when I had started my career as a process engineer in charge of sampling new molds and developing the molding processes, I found that our engineering team was doing repetitive calculations and tasks. I had developed a method to calculate shrinkages before the mold was built based on the data I had on hand. I had a lot of data because we molded automotive parts and used the same grades of nylon and PBT for most parts. The formulas were built on some empirical background and knowledge. I used to call this project ‘Experienced Molding.’ As several industries started thinking along these lines and seeing the potential benefits, the terms AI and ML were born. The exponential increase in computing power has also helped the rise of these technologies.
AI gives computers the ability to think and reason like humans do and address problems logically. ML is a subset of AI. It enables computers to do their work without specifically writing a program to perform the task.
Supervised and Unsupervised Learning
Historically, cleverly written computer programs did all the work. Computers have been programmed by programmers to do complex calculations and to accurately predict results of scientific and engineering phenomena. Domain experts have contributed their knowledge gathered over years into algorithms that do the work. Machine Learning is the science that makes computers work for you without being specifically programmed to do so. Predictive models based on Machine Learning are now working in spheres of business, science, entertainment and many more to deliver results with accuracy and efficacy that were never seen before. Machine Learning methods have evolved over the years. These methods are broadly classified into two categories: supervised learning and unsupervised learning.
Supervised learning provides labels, which means it provides data that includes potential causes as well as outcomes that go into learning. For example, a labeled data could be a data set that includes melt temperature, melt viscosity and injection speed, which are inputs or factors of the molding process, as well as the associated outputs or responses such as part dimensions and cosmetics. Examples of cosmetics would be the presence or absence of flash, sink or splay.
A supervised learning algorithm will observe and analyze the data set and figure out the underlying model which is related to the molding process inputs and their interactions with the outcome (such as part dimensions). The method described here is a Regression method where you build a predictive model that predicts numerical values. Other supervised learning methods may aim at classification of outcomes. They could classify outcomes into acceptable parts and nonacceptable parts, or parts with sink marks or without.
Unsupervised learning does not use labels. The algorithms seek to find similarity in multiple attributes and cluster data points together using these attributes. Here, the effort is to find attributes that behave similarly and work upon them with a common strategy. For example, a clustering algorithm may find that youngsters in geographical regions with certain weather conditions are more interested in competitive sports. Sports goods and dietary supplement advertisements are then focused more sharply on these regions.
Deep Learning
The last decade saw the dominance of the Deep Learning technology, which is a category of ML. It uses Artificial Neural Networks (ANN) to emulate the human brain. A human brain has millions of neurons that are connected together through synapses. Each neuron takes in a piece of information and makes a tiny unit decision. The unit decisions put together result in the human response. The ANN equivalent of a neuron is a perceptron. A perceptron is a tiny computer program that performs a unit mathematical function (called activation function) that transforms input signals into outputs. Refer to Figure 1.
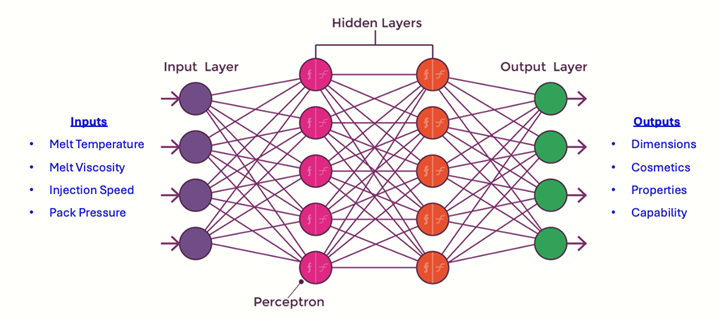
In a fully connected network, perceptrons are arranged in layers, and each layer is fully connected to the next one. Source: FimmTech
In a fully connected network, perceptrons are arranged in layers, and each layer is fully connected to the next one. An ANN has an input layer that takes in the signal, which is then processed by the intermediate hidden layers that have mathematical activation functions, weights and biases. The Output layer produces the predicted results.
A Neural Network is trained on individual processes by showing it a data set that contains predictors and label values. These are inputs and outputs. In the example we saw earlier, the predictors were melt temperature, melt viscosity and injection speed, while the label was the part dimension. The ANN learns by observing many thousand data points and fine-tuning itself. The ANN that is thus trained is now capable of predicting with a high degree of accuracy and speed.
Neural Networks have evolved into Convolutional Neural Networks (CNNs) that deal specifically with image processing that can be used to detect cosmetic defect in molded products.
Whether one is aware or not, everyone is already immersed in AI and ML. Take for example, Google Maps. Google Maps can now predict the exact number of minutes it will take you to reach your destination. It can do so because it has ‘learned’ from all the times that all the drivers journeyed on that route at all times of the day. It knows it will take more time in the morning, Monday through Friday, because of the traffic from people going to work.
It has that data for multiple years, and the predictions get better and better because it is collecting even more data every day and using the feedback to modify the model on a continuous basis. Here some of the predictors are time of day, day of the week, traffic density and weather, while the labels are time of arrival, time it takes to reach the destination and the suggestions, if any, to present alternative routes. It is an intelligent system that no human needs to be involved with and is therefore called Artificial Intelligence.
Although we have begun to understand the science of injection molding to deeper levels in the last few years, there are still a lot of unknowns. For example, how does one calculate the tonnage required for a particular part before the mold is even designed? In the November 2023 issue of PT, I described the challenges in detail. Similarly, predicting the other process parameters such as injection pressures, pack-and-hold pressures and cooling times are challenges. Simulation software has improved significantly, but we frequently hear, “The actual pressure is not what we see in simulation results,” or something similar. This is where AI and ML can play a role and help molders predict more accurately and perhaps someday be able to come very close to the actual. All we need is the inputs from the past billions of molds built and the processes used to mold parts with them. Of course, that is not possible, but a start can be made with what is on hand, and the data can be continuously fed as more and more molds come into the molding facility.
An initial effort was started by my company, FimmTech Inc., Carlsbad, California. A custom online portal was built on a test basis. The goal was to build a model for predicting the required tonnage for a given mold, and data from a few molds was used. The part projected area, part thickness, flow length from the gate, peak injection pressures and pack pressures were recorded for each mold. The pack pressure applies the pressure on the part projected area affecting the tonnage. The flow length is one of the factors for pressure drop and therefore affects the end-of-fill pressures, such that the thicker the part, the higher the required packing pressures. This is the theory we know, but we must consider the different interactions and interdependencies of the inputs on the outputs. This is where ML helps. A model was developed with limited data, and the program was able to closely predict and calculate the required tonnage for a mold that was in production. The tonnage was optimized earlier based on the Tonnage Study.
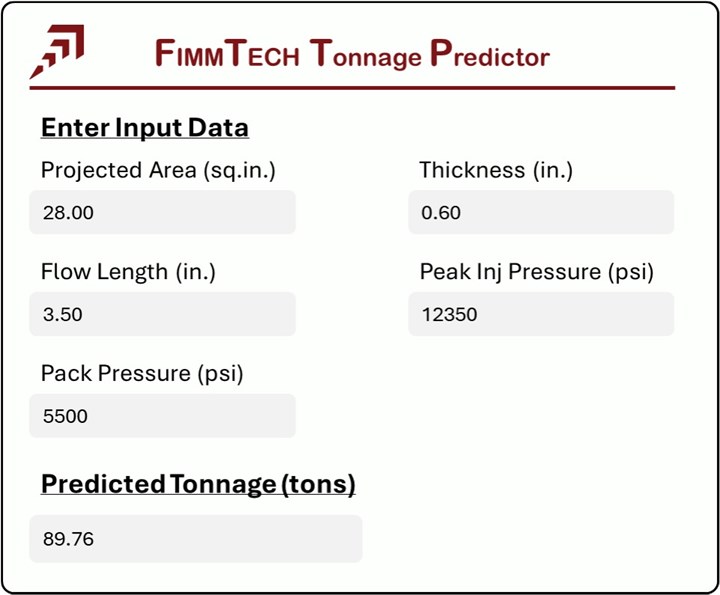
Input parameters for the software and the respective outputs after calculations. Source: FimmTech
Figure 2 shows the input parameters for the software, and the related output after the calculations. This is a mockup example where the part projected area is 28 in2; the part thickness is 0.60 in. and the distance from the gate to the end of fill is 3.5 in. The peak pressure used by the machine was 12,350 psi with a packing pressure of 5,500 psi. With this information, the software predicted the tonnage to be 89.76 tons.
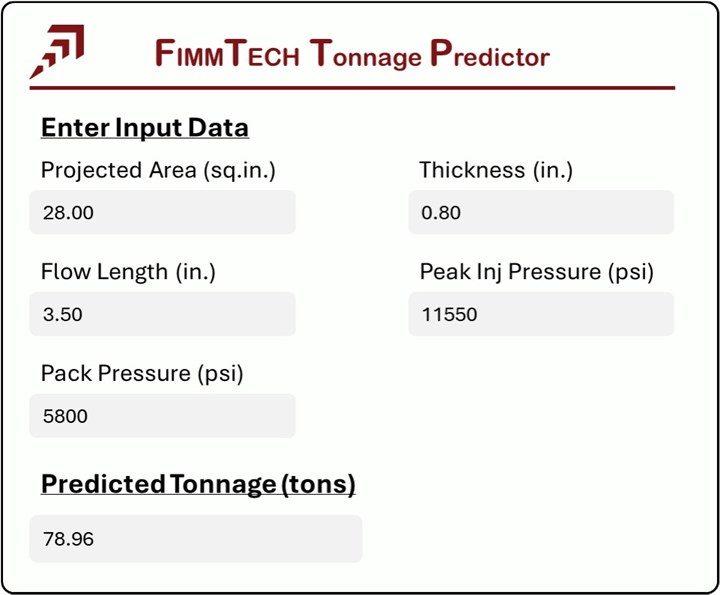
As the inputs change, the calculations change. Source: FimmTech
Figure 3 shows the same part but with an increased part thickness of 0.80 in. from 0.60 in. The peak pressure was lower and the pack pressure was higher. The predicted tonnage was 78.96 tons. Figure 4 shows another example with a prediction of 101.52 tons.
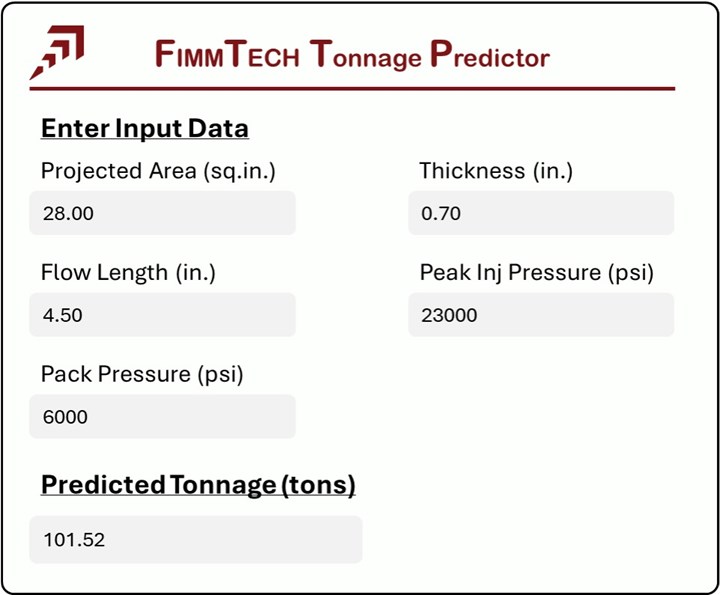
Tonnage required predicted on the basis of five different data points. Source: FimmTech
Scientific molding deals with a lot of theory, all of which is relevant and important. Using AI and ML techniques, we get closer to the real predictions. The biggest hurdle is getting all the initial data from the part design, material, mold, machine and process. The richer the data, the better the prediction will be. The predictions do not have to be restricted to tonnage as shown above. A complete molding process can be predicted if a part and mold are described to the program. Simulation programs do similar predictions, but those are based on established algorithms built on the theories of rheology, heat transfer, thermodynamics and related sciences. Simulation programs have become very powerful over the years and are now also beginning to incorporate AI and ML into their predictions. This is very good news for the molding industry because all these technologies will soon help us become a better and more efficient industry.
ABOUT THE AUTHOR: Suhas Kulkarni is the founder and president of FimmTech, San Diego, an injection molding service-oriented firm focusing on Scientific Molding. FimmTech has developed several custom tools that help molders develop robust processes, and its seminars have trained hundreds of individuals. Kulkarni is an author of the best-selling book, “Robust Process Development and Scientific Molding,” published by Hanser Publications. Contact: 760-525–9053; suhas@fimmtech.com; fimmtech.com.
Related Content
The Importance of Barrel Heat and Melt Temperature
Barrel temperature may impact melting in the case of very small extruders running very slowly. Otherwise, melting is mainly the result of shear heating of the polymer.
Read MoreInjection Molding: Focus on these Seven Areas to Set a Preventive Maintenance Schedule
Performing fundamental maintenance inspections frequently assures press longevity and process stability. Here’s a checklist to help you stay on top of seven key systems.
Read MoreWhy Shoulder Bolts Are Too Important to Ignore (Part 1)
These humble but essential fasteners used in injection molds are known by various names and used for a number of purposes.
Read MoreThe Effects of Stress on Polymers
Previously we have discussed the effects of temperature and time on the long-term behavior of polymers. Now let's take a look at stress.
Read MoreRead Next
Video: Prepping Better Melt Preparers
The Molding 2022 conference concluded with a special half-day workshop and tour at the Polymers Center of Excellence.
Read MoreClamp Tonnage: More Is Better...Right?
Determining the correct tonnage provides a foundation that must be rock-solid to avoid flash and damage. But applying excessive force can create problems with the part, mold, and machine.
Read MoreIs There a More Accurate Means to Calculate Tonnage?
Molders have long used the projected area of the parts and runner to guesstimate how much tonnage is required to mold a part without flash, but there’s a more precise methodology.
Read More